When people hear the term "Artificial Intelligence," they mostly think of ChatGPT. Sure, Generative AI tools are impressive. But it’s only one part of a much larger story. AI is far-reaching, with applications and methods that shape industries in ways many people don’t realize.
Inspired by Jeff Winter's thoughts on AI's taxonomy, let's dive deeper. Generative AI might be the headline act, but the entire AI ecosystem is where the real magic happens.
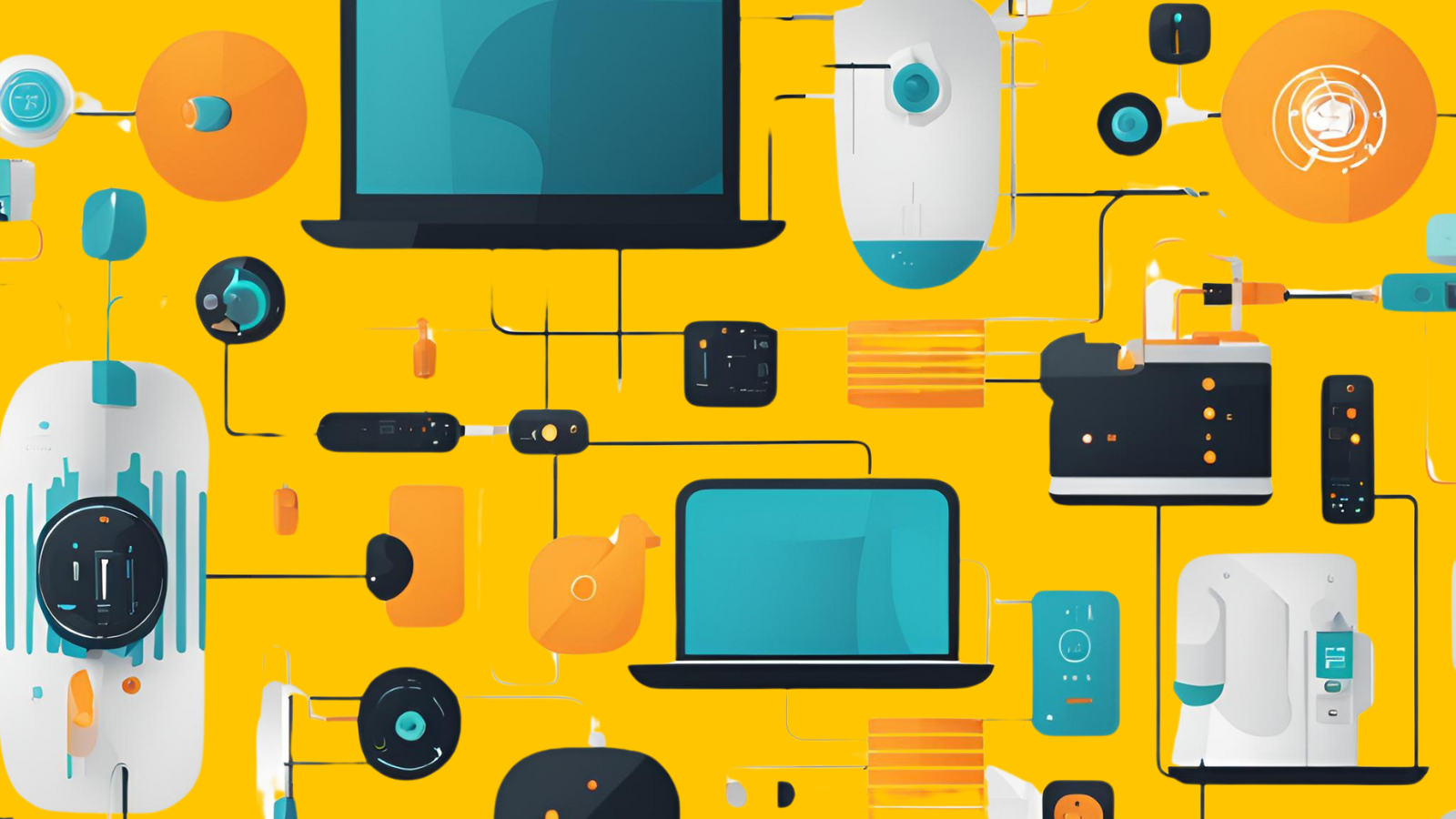
AI Is Always Changing
One of AI’s coolest features? It doesn’t sit still. AI evolves constantly, adapting to solve new challenges. As Jeff Winter puts it, “There is no universally agreed-upon definition” for its subfields. That fluidity is what keeps it exciting.
At its core, AI is about systems that mimic human thinking. Whether it’s problem-solving, decision-making, or learning, AI mimics what we do — but faster and often better. Think of it as an umbrella covering different approaches. Beneath it, you’ll find everything from rule-based systems to advanced neural networks.
Symbolic AI: The Early Days
Symbolic AI is where it all started. These rule-based systems follow strict “if-then” instructions to get things done. They’re reliable for well-defined tasks like analyzing contracts or diagnosing straightforward medical conditions.
Take MYCIN, an early medical AI program. It helped doctors diagnose bacterial infections by following precise rules. Symbolic AI might not adapt well to new situations, but in structured environments, it shines.
Machine Learning: Where AI Learns
Then came Machine Learning (ML), and everything changed. ML taught AI to learn from data instead of relying on hard-coded rules. This made AI smarter and more flexible.
ML comes in three main flavors:
- Supervised Learning: Think of it as teaching by example. Feed it labeled data (like pictures of cats and dogs), and it learns to recognize them. It’s used for things like fraud detection or predicting customer behavior.
- Unsupervised Learning: When there are no labels, ML looks for patterns. Businesses use it to group similar customers or detect equipment issues.
- Reinforcement Learning: This is trial and error on steroids. The AI learns by interacting with its environment and figuring out what works. It’s great for optimizing supply chains or teaching robots to navigate spaces.
From Netflix recommendations to smarter logistics, ML powers so much of what we rely on today.
Deep Learning: The AI Powerhouse
Deep Learning, a subset of ML, takes AI to the next level. It’s like a brain-inspired system that processes massive amounts of data to uncover patterns.
But Deep Learning isn’t just for Generative AI. It also powers:
- Self-driving cars that process traffic data in real time.
- Medical imaging to detect diseases like cancer earlier and more accurately.
- Voice assistants like Alexa and Google Assistant.
Deep Learning has become the backbone of modern AI. Its applications stretch far beyond text generation or image creation.
Generative AI: The Buzzword
Generative AI is the cool kid on the block right now. Tools like ChatGPT and MidJourney have made AI fun and approachable. These tools generate text, art, and even music.
But here’s the deal: Generative AI is only one part of the puzzle. The rest of AI quietly solves problems behind the scenes:
- Fraud detection models flag unusual activity.
- Optimization algorithms make supply chains faster and more efficient.
- Anomaly detection keeps factories running smoothly by spotting issues early.
Generative AI might grab headlines, but it’s just one chapter in the AI story.
How AI Shapes the World
AI is more than a novelty. It’s transforming industries every day. Here are a few examples:
- Healthcare: AI analyzes patient data, predicting diseases before symptoms show up. IBM Watson, for instance, helps doctors decide on treatments.
- Retail: Ever wonder why Amazon knows exactly what you want? AI-powered recommendation engines make that happen.
- Energy: Smart grids use AI to balance electricity demand and supply, cutting waste and saving money.
- Agriculture: Farmers use AI to monitor crops and decide the best time to harvest.
AI isn’t just changing the future; it’s already shaping our present.
Why It’s More Than Generative AI
It’s easy to focus on the creativity of Generative AI. But doing so misses the bigger picture. AI does so much more than generate — it solves, optimizes, and predicts.
Think about what businesses really need:
- Scalability: Predictive models help companies grow efficiently.
- Stability: Rule-based systems and anomaly detection keep operations running smoothly.
- Innovation: AI insights unlock new markets and opportunities.
By looking at the full AI spectrum, businesses can unlock its real power.
Connecting the Dots
Jeff Winter’s taxonomy highlights the overlap between AI’s branches. For example:
- Symbolic AI’s rule-following precision works well with Machine Learning’s adaptability.
- Generative AI can create synthetic data to train predictive models faster.
- Reinforcement learning fine-tunes operations in real-time, while Deep Learning ensures accuracy.
It’s not about choosing one approach — it’s about combining them to solve complex problems.
Lessons for Startups
For startups, the takeaway is clear: Don’t get caught up in the Generative AI hype. It’s exciting, but it’s not the whole story. Ask yourself:
- What problem are we solving?
- Which AI approach fits best?
- How can we use multiple AI methods to get the best results?
AI isn’t a one-size-fits-all solution. By thinking strategically, you can find the perfect fit for your business.
Beyond the Buzz
Generative AI is amazing, no question. But it’s just a small part of what AI can do.
From rule-based systems to predictive models, the AI ecosystem is vast and varied. By exploring the full spectrum, we can harness AI’s true potential to transform industries and solve real-world problems.
AI isn’t just about what machines create — it’s about how they learn, adapt, and improve our world. So let’s look beyond the buzzwords and dive deeper into what AI can truly achieve.
Why Startups Need More Than Generative AI
Generative AI gets all the attention, but it’s not the full story. The AI ecosystem offers tools for every stage of business growth. Startups can’t afford to overlook these:
- Operational Intelligence: Machine learning (ML) models can predict operational bottlenecks, optimize logistics, and save costs by analyzing patterns in real-time.
- Customer Understanding: Unsupervised learning clusters customer data to reveal insights like preferences or churn risks. This helps startups tailor experiences and retain users.
- End-to-End Automation: From AI chatbots to automated invoicing systems, symbolic AI and reinforcement learning can streamline repetitive processes, saving time and resources.
These applications aren’t just cool — they’re practical. They help startups build efficiency and resilience into their operations.
Where Generative AI Fits In
Generative AI is excellent at tackling creative and customer-facing tasks. But to make the most of it, you need to integrate it into broader workflows.
Here’s how startups can use Generative AI alongside other AI tools:
- Customer Support: Tools like SAP’s Joule categorize emails, analyze sentiment, and suggest next steps, speeding up response times.
- Content Creation: Pair Generative AI with predictive models to personalize marketing campaigns at scale.
- Data Summarization: Use foundation models to quickly analyze and summarize reports, enabling data-driven decision-making.
When paired with operational AI, Generative AI becomes even more powerful, helping startups create, act, and optimize simultaneously.
Why Generative AI Still Needs Support from Other AI Tools
Generative AI can produce amazing outputs, but it’s not great at verifying its own work. That’s where other AI tools step in:
- Symbolic AI: Still useful for structured tasks like compliance checks or rule-based automation.
- Predictive Models: Help identify risks, trends, and opportunities in real-time.
- Orchestration Tools: Combine multiple AI models to tackle complex workflows. For instance, SAP’s Generative AI Hub integrates models like GPT-4 with enterprise tools for better outcomes.
Conclusion
By using the right mix of tools, startups can go beyond content generation and tackle operational and strategic challenges head-on.